False Positives: 3 Ways Artificial Intelligence Is Easing The Perpetual KYC/AML Headache

Let’s start with some definitions and stats to set the scene.
What Are False Positives In A KYC/AML Context?
False positives are legal and lawful transactions or customer profiles that are flagged as suspicious by a company’s monitoring system. As a result, the transaction, account or profile associated with a customer is blocked and/or suspended.
What follows a suspicious activity report (SAR) is the long and arduous process of manual reconciliation. Banks spend an enormous amount of time, money and internal resources to verify whether these alerts are indeed something to worry about.
61% of finance organizations are “highly dependent” on spreadsheets, according to an IMA survey. We live in a digital era and depending on manual processes is a serious problem.
The False Positive Problem: Just How Bad Is It?
Here’s a few highlights from a recent Celent white paper:
- “A flood of false positives and heavy reliance on manual processes are making AML programs costly, inefficient, and unsustainable.”
- Banks typically report 90–99% of all alerts are false positives, while those with advanced tuning capabilities report a lower, but still high figure of 80–85%.
Then you have this incredible stat by IBM:
- As much as 90% of notifications about potential suspicious activities do not result in the filling of a suspicious transaction report.
KPMG adds more reasons for concern by reporting that:
- Despite recent advancements and investments in new technology, 51% of banks still reported a high rate of false-positives, resulting from their technology solutions, decreasing efficiencies in fraud detection.
As you can see, this is not merely a problem, it’s a plague. FIs are caught between their attempt to follow the letter of the law, protect customers and their own brands, and untangle an operational nightmare.
Enter artificial intelligence.
AI is the new frontier to the problem of false positives and today we will examine 3 ways it is going to revolutionise the AML/KYC space.
Before we dive into it, here’s a 2017 article by McKinsey, foreseeing this change:
“...banks should invest in three areas: efficient data-aggregation platforms, advanced statistical modeling (such as machine learning-based risk scoring and alert-generation engines), and automation of processes (such as investigator visualization tools).
“...false-positive alerts can be brought down from over 90 percent to below 50 percent.”
Now that we have identified both the concept and impact of false positives, let’s look at the solutions.
Identifying Customer Behavior Changes
Traditional systems use rules-based transaction monitoring solutions. While the rules are configurable, they are not particularly proactive in analysing data and distilling useful insights.
On the contrary, artificial intelligence has the ability to develop models that track customer behaviour in real time, build specific profiles and identify changes that are deemed to be irregular.
The system won’t flag a transaction based on a number-threshold but rather on whether it fits the profile and behaviour of the customer in question.
The most fascinating element about this approach is that the algorithm will keep on learning, becoming more “knowledgeable” as time goes by. The more transactions a customer completes, the more data for the algorithm to make its alert more accurate.
Harnessing Unstructured Data
Verifying the identity of the new customer is simply the first step of the KYC process. In order to build a comprehensive customer profile before onboarding a new client, companies need to search for and collect a diverse range of information.
Here is the most common background information and data companies look to collect:
- Professional
- Political
- Social
- Institutional
- Financial
The obvious question that comes to mind is, where do they get this information from? Again, the job of harnessing this data is difficult as it is scattered around in different places.
- Online and offline media
- Public archives
- Social networks
- Open-source data sources
- Firewall libraries
- Paid subscription directories
- Government portals
While locating and gathering this information is a tall task, it is actually not the hardest part of the process. Discovery is only the tip of the iceberg for companies that if and when they do get their hands on this data, they need to make sense of it.
They need to draw connections, come to meaningful conclusions, connect the dots and distill the meaning from the unstructured data they just gathered. This is where artificial intelligence and natural language processing (NLP) come into play.
It is the technology that finally gives companies the insights they had to manually had to deduct for themselves all these years. It cross-references information from all these different sources, automating the building of a true risk-based profile for each customer.
By managing to mirror the most up-to-date profile for each client, companies can decrease their exposure to false positive alerts. They are in the unique position to know the risk-level attached to each entity and validate the truthfulness of the alerts that come from their activity.
Learning, Adapting, & Responding
Much like NPL, machine learning is yet another subset of artificial intelligence that will play a huge role in battling false positives in the near future.
Machine learning has the distinct advantage of creating unique fraud detection systems. This allows the technology to make educated predictions without being explicitly programmed to perform a specific task.
What the program essentially does is estimate the behaviour of an entity, anticipating a certain action. If the action falls way outside the predicted framework, the technology can then begin to assess the risk factor associated with the entity.
Takeaways
Is this actually working or is it just a beautiful idea that can’t be implemented?
Well, if numbers are anything to go by, machine learning is actually producing results. A case study from Teradata showed that the implementation of machine learning reduced false positives by 60%, and was expected to rise to 80% as the model continued to learn.
That’s both impressive and promising. Artificial intelligence is revolutionizing the entire KYC/AML industry one but at a time. At Identomat, we leverage the powers of AI on a daily basis when building our proprietary technology.
We understand the value it brings to your company and we strive to stay on top of the latest advancements and developments in the field. If you have any questions about the technology, our solutions or the ways it can help your company, do not hesitate to reach out and inquire.
Our team is always ready and willing to give you the answers and solutions you’re looking for.
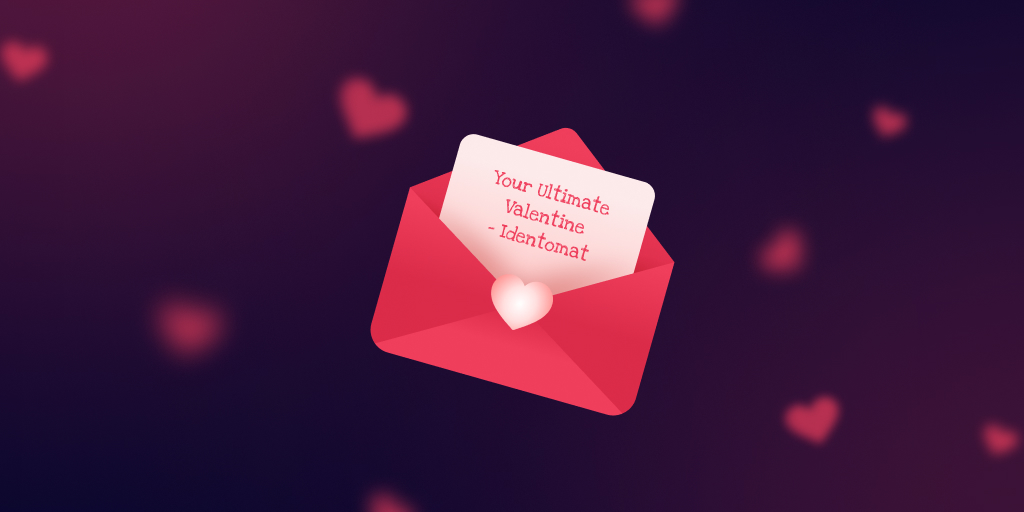
Valentine’s Day 101: Swipe Right, Verify Twice By your ultimate Valentine - Identomat!
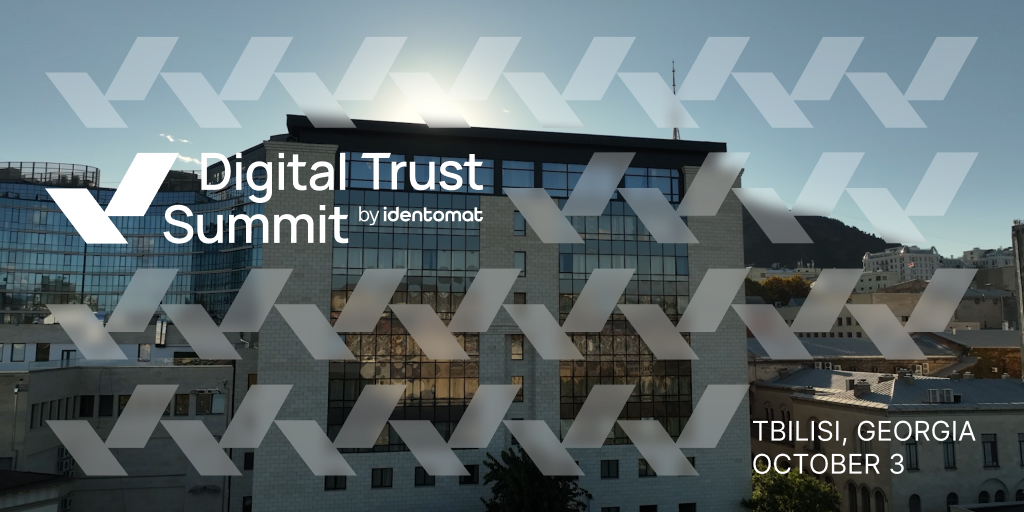